What is the Regression Discontinuity Design and How Can it be Used to Evaluate Crime and Justice Interventions?
Posted on
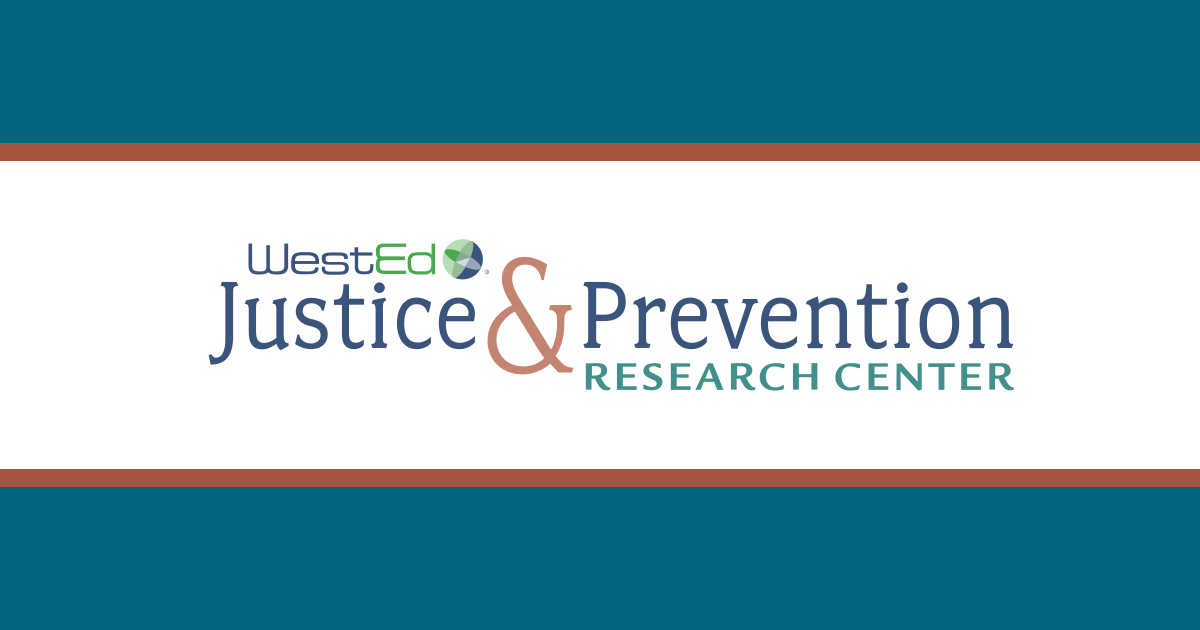
By Jonathan Nakamoto, Trent Baskerville, and Anthony Petrosino of the Justice & Prevention Research Center at WestEd and Alexis Grant from the Resilient and Healthy Schools and Communities team. This article was originally published by George Mason University’s Center for Evidence-Based Crime Policy in the spring 2024 edition of Translational Criminology.
There are many ways to evaluate an intervention, program, or policy to see if it works. It would be wonderful if all of these different approaches, or “research designs,” came to the same conclusion. In an ideal world, our approach to evaluating a program would not matter. However, it turns out research is not so simple. The results we observe can often be confounded by, or due to, the evaluation design we use to determine whether something worked. A weak design could result in a false positive, in which an ineffective intervention is incorrectly credited with a good outcome. The converse is also true: a weak design could result in a false negative, in which the intervention is wrongly determined to have been unsuccessful.
Fortunately, researchers have been toiling for decades on developing and promoting more rigorous methods. The most commonly known approach to increase our confidence and reduce our skepticism is a randomized controlled trial (RCT), in which individuals or groups of individuals (e.g., prison units, neighborhoods) can be assigned using the play of chance (randomization) to either receive an intervention or to a control group that does not receive an intervention.
Another such approach, which is less well known, is the Regression Discontinuity Design (RDD). We reviewed the crime and justice literature to examine the prevalence of RDD studies and found that it has been used less often than other methods, such as the RCT. In this brief, we provide an overview of RDD, including what it is and why it is a powerful approach to evaluation. We highlight one example and conclude with a call to action to promote its greater use.
What is Regression Discontinuity Design (RDD)?
RDD allows us to examine the impact of an intervention when individuals or groups are assigned to the treatment and control conditions solely based on a cutoff threshold on a numeric score. In such situations, entities scoring above the cutoff receive treatment and those who score below it do not. These numeric scores can come from any type of data. For example, towns that are assigned to implement a new violence prevention initiative based on exceeding a certain violent crime rate would be one example. Every town above the rate would get the program; every town below the rate would not get it. Another example is assigning incarcerated persons to specific treatment based on a classification score upon intake.
You might ask why is using this numeric score so important to the strength of the RDD? It has to do with how it creates a comparison group. And it has to do with the entities that score just above or below the cutoff. Let’s see if we can better illustrate the strength of RDD using a hypothetical example. Let’s use a risk assessment score for assigning youth at high risk for violence treatment services; persons scoring 75 or higher are deemed high risk and will receive treatment.
We can assume that someone who scores a 99 and someone who scores a 43 are vastly different in their risk levels. But what about the people who score 74 and those who score 75? We would assume that the level of their risks and needs are very closely matched. Although they are assumed to be similar, one will receive treatment, and the other will not. RDD exploits this cutoff rule. Since we can assume the individuals are quite similar just above and below the cutoff, we can also confidently assume that the difference between the outcomes for entities just above the cutoff compared to those just below the cutoff provides a valid estimate of the impact of the intervention. Researchers would argue that these estimates from RDD are at the high end of causal inference, and we can be more confident about the observed results.
Another benefit to the RDD is that it can be visually compelling. In our example, if there is a positive treatment impact, youth just above the cutoff should do better on criminal offending outcomes than youth just below the cutoff. We should see a “discontinuity” or “break” in the expected outcomes. If there is no program impact, there likely would be no “discontinuity” or “break.”
The figure above indicates that the program was successful. There is a discontinuity or break, with the youth just above the cutoff (who received the program) committing fewer new offenses at the end of one year than youth just below the cutoff (who did not receive the program).
Our goal in this article is not to go in-depth into the technical details of RDD. We also do not want to make RDD seem so easy that there are no challenges to implementing it. RDD is fairly straightforward but technical details related to RDD planning and analysis will require a methodologist with experience with the design. There are many excellent resources to guide its use.
What About an Actual Example of How RDD Was Used to Study a Justice Policy?
It is challenging to construct a study to better understand the impact of prison. Most persons sentenced to prison commit offenses that are more serious than those who receive alternative sanctions such as probation. However, Mitchell and his colleagues found an innovative way to do so using RDD. They took advantage of a large historical database in Florida that had over 262,000 individuals convicted of felonies and their sentences. It turns out that Florida assigns points at sentencing, known as “total sentence points.” How these points are assigned in Florida is based on several factors including the seriousness of the offense and the defendant’s prior criminal record. Cases with more than 44 total sentence points are “scored to prison” and cases with 44 or fewer points receive probation, jail, and/or house arrest.
Like every design, RDD can face some challenges in the field. One of those challenges is that even if a score like total sentencing points is solely used to determine whether a person gets prison or not, there can be slippage. In Florida, judges are given considerable leeway to override this assignment. And, further complicating matters, it turns out that these overrides happen quite often: 13% of cases just below the cutoff still received prison sentences (when they should have gotten alternative sanctions), and only 39% of cases just above the cutoff actually received prison sentences (meaning 61% who should have gotten prison just above the cutoff did not).
Technically speaking, when there is slippage like this, researchers refer to the RDD as being “fuzzy.” However, even with this fuzziness, the researchers argued that there is a sufficient sample at the cutoff to allow for valid conclusions to be drawn. Mitchell and his colleagues did a lot of complex analyses, but the overall message was this: For cases near the cutoff, there is no evidence that prison sentences led to reductions in subsequent recidivism over a three-year period.
How Can Policymakers, Justice Leaders, and Researchers Use RDD?
We believe that policymakers (e.g., agency leaders), practitioners, and researchers should consider the use of RDD because it allows for stronger conclusions to be drawn about the impact of an intervention than several commonly used research designs in the field.
We believe that policymakers (e.g., agency leaders), practitioners, and researchers should consider the use of RDD because it allows for stronger conclusions to be drawn about the impact of an intervention than several commonly used research designs in the field (e.g., the pre-post or before and after design, the non-equivalent comparison group design). RDD is very well suited for many situations in the crime and justice area because the highest need persons, areas, or entities often deliberately receive treatment. In most instances, we would be concerned about the bias of that approach, that persons are being deliberately selected and assigned to treatment. But in this instance, if a numeric score and threshold (i.e., the cutoff) are used to assign the intervention, we can turn that bias around and use it in a powerful way in RDD to increase causal inference and our confidence in the findings.
We urge agency leaders to prospectively plan RDD studies with researchers. An example would be when an evaluation is needed for a particular program. Let’s say it is for a treatment program for high-risk people. The agency leaders and practitioners could collaborate with researchers to identify an existing instrument for classifying risk or develop a new one. In some cases, minor changes to existing practices (e.g., developing a more formalized risk assessment system) would allow for the use of RDD. Policymakers and practitioners could also collaborate with researchers to identify the cutoff score they would be comfortable with, that those above would receive treatment and those below would not.
Planning an RDD prospectively in this way can have several benefits, such as selecting the right factor to assign entities (the assignment variable) with enough variation in scores (e.g., we would not use a 1-2-3 scale), selecting the best cutoff threshold that is not too high or low (e.g., a scale of 1-100 that assigns only those scoring over 95 to treatment), stressing the importance of limiting fuzziness (e.g., overrides to the cutoff threshold), and paying attention to sample size (RDD can require a much larger sample size than other designs including the RCT). But, when prospective studies cannot be done, let’s not forget that most RDD studies have been done retrospectively. In our review of RDD studies in crime and justice, we have found that nearly all of them are retrospective. In the Florida study described above, researchers used existing data and were able to distinguish between entities receiving the intervention or not (i.e., the cutoff threshold), and analyze the impact for these groups on selected outcomes (crime or recidivism).
Retrospective studies are also advantageous as they can be cheaper than prospective studies in the field (as the data have already been collected). They are also less obtrusive—the researchers can do the analyses without bothering anyone outside the research team! However, it is critical that researchers be granted access to these data, and that they include, at minimum, the assignment variable (e.g., if age is the assignment variable, the age of each person is available in the data set) and the outcomes of interest (e.g., recidivism). But retrospective analyses such as the Florida example can yield important insights to guide crime and justice policy.
Conclusion
Although the number of published studies that use RDD in the crime and justice field has been growing in recent years, it is still quite low relative to how many evaluations have been published since RDD was first popularized in the 1960s. Our preliminary review of the literature identified less than 70 available RDD studies. We call more attention to the design and encourage its wider adoption whenever possible to improve our claims about the effectiveness of programs and policies to reduce crime and improve the justice system.
Authors’ Note
Portions of this article are also published as a research brief.