Making Sense of Temporal Data with an Intelligent Tutoring System
Posted on
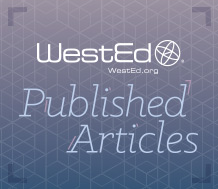
Article: A Novel Method for the In-Depth Multimodal Analysis of Student Learning Trajectories in Intelligent Tutoring Systems
Publication: Journal of Learning Analytics, a peer-reviewed journal
Publisher: University of Technology Sydney ePRESS
Publication Date: April 9, 2018
Related WestEd Author: Jodi Davenport
Related WestEd Program: Science, Technology, Engineering, & Mathematics
Abstract:
Temporal analyses are critical to understanding learning processes, yet understudied in education research. Data from different sources are often collected at different grain sizes, which are difficult to integrate. Making sense of data at many levels of analysis, including the most detailed levels, is highly time-consuming.
This article describes a generalizable approach for more efficient yet rich sensemaking of temporal data during student use of intelligent tutoring systems. This multi-step approach involves using coarse-grain temporality — learning trajectories across knowledge components — to identify and further explore “focal” moments worthy of more fine-grain, context-rich analysis.
The authors discuss the application of this approach to data collected from a classroom study in which students engaged in a Chemistry Virtual Lab tutoring system developed by The ChemCollective group at Carnegie Mellon University and WestEd’s Science, Technology, Engineering, & Mathematics program.
The authors also show that the application of this multistep approach efficiently led to interpretable and actionable insights while making use of the richness of the available data. This method is generalizable to many types of datasets and can help handle large volumes of rich data at multiple levels of granularity. They argue that it can be a valuable approach to tackling some of the most prohibitive methodological challenges involved in temporal learning analytics.